Introduction
In an increasingly digital world, the sophistication and volume of cyber threats have grown exponentially. Organizations face a myriad of challenges—from data breaches and ransomware attacks to phishing scams and insider threats. Traditional security measures, often reliant on static rules and signature-based detection, struggle to keep pace with these evolving threats. This is where Artificial Intelligence (AI) and Machine Learning (ML) come into play, offering dynamic, adaptive solutions that enhance an organization’s ability to prevent, detect, and respond to cyber threats in real-time.
Defining AI and Machine Learning
To fully appreciate the role of AI and ML in cybersecurity, it’s essential to understand these concepts:
- Artificial Intelligence (AI): This encompasses the simulation of human cognitive functions by machines. AI enables systems to perform tasks that typically require human intelligence, such as reasoning, learning, problem-solving, and understanding natural language.
- Machine Learning (ML): A subset of AI, ML focuses on the development of algorithms that allow computers to learn from and make predictions based on data. Instead of being explicitly programmed for each task, ML algorithms use statistical techniques to improve their performance as they are exposed to more data.
The Transformative Impact of AI and Machine Learning on Cybersecurity
1. Enhancing Threat Detection Capabilities
a. Advanced Behavioral Analytics
AI and ML excel at analyzing user behavior patterns. By establishing a baseline of normal activities, these systems can identify deviations that may indicate potential threats. For example, if a user typically accesses the company network from a specific geographical location but suddenly logs in from a different continent, an alert can be triggered for further investigation.
Example: Companies like Darktrace use AI-driven behavioral analytics to detect anomalies in network traffic. Their self-learning technology continuously adapts to the unique behavior of every user and device within a network, allowing for real-time identification of suspicious activities.
b. Predictive Threat Intelligence
Predictive analytics powered by AI can analyze historical data to identify patterns and trends. This allows organizations to anticipate potential cyber incidents before they occur. By understanding how attacks have evolved over time, businesses can fortify their defenses accordingly.
Example: IBM’s QRadar uses AI to provide predictive insights into potential security threats, enabling organizations to stay one step ahead of cybercriminals.
2. Automating Incident Response
a. Immediate Threat Mitigation
AI systems can automate responses to detected threats, reducing the time it takes to respond and mitigate potential damage. For instance, if malware is detected on a network, an AI-driven system can immediately isolate the infected device, preventing further spread.
Example: Solutions like Splunk and Palo Alto Networks offer automated incident response capabilities, allowing organizations to respond to threats in a matter of seconds rather than minutes or hours.
b. Streamlined Incident Management
AI can assist in developing and refining incident response playbooks by analyzing historical incidents and suggesting tailored strategies for future threats. This data-driven approach enhances an organization’s preparedness and response efficiency.
Example: Microsoft Azure Sentinel uses AI to correlate alerts from various sources, enabling security teams to focus on the most critical incidents and streamline their response efforts.
3. Phishing and Social Engineering Defense
a. Intelligent Email Filtering
Phishing remains one of the most common attack vectors. AI algorithms can analyze email content, sender behavior, and recipient interactions to identify and filter out phishing attempts effectively. By scrutinizing the language used in emails and the metadata associated with them, AI systems can flag suspicious messages before they reach the end-user.
Example: Barracuda Networks employs AI-driven email protection solutions that continuously learn from both historical and real-time data to identify and block phishing attempts with high accuracy.
b. URL and Domain Analysis
AI and ML can analyze URLs and domains in real time to detect malicious activity. By evaluating factors such as domain reputation, historical behavior, and user reports, these systems can prevent users from visiting harmful sites.
Example: Cisco’s Umbrella uses DNS-layer security to provide intelligent URL filtering, blocking access to domains associated with known threats based on real-time data.
4. Proactive Malware Detection
a. Behavioral Analysis Over Signature Matching
Traditional malware detection methods often rely on signature-based detection, which can miss new and unknown threats. AI systems, on the other hand, can analyze the behavior of files in isolated environments (sandboxing) to identify potential malware based on their actions rather than their known signatures.
Example: CrowdStrike’s Falcon platform employs advanced behavioral analysis to detect and respond to threats by monitoring the actions of files in real-time, providing protection against both known and unknown malware.
b. Continuous Model Improvement
Machine learning models improve over time by learning from new malware samples. This continuous learning process ensures that detection capabilities remain robust against emerging threats. As new malware variants are released, AI systems can quickly adapt, enhancing their effectiveness.
Example: Google’s VirusTotal leverages machine learning to analyze and classify files and URLs. As the system encounters new threats, it continuously updates its models to improve detection rates.
5. Fortifying Network Security
a. Intelligent Intrusion Detection Systems (IDS)
AI-powered Intrusion Detection Systems (IDS) analyze network traffic in real time, identifying unusual patterns that may signal unauthorized access attempts. These systems can differentiate between benign anomalies and genuine threats, allowing for prompt action.
Example: Anomaly detection solutions like Sumo Logic utilize AI to monitor network traffic and detect potential intrusions by learning what constitutes normal behavior for each network segment.
b. Anomaly Detection Techniques
Machine learning algorithms can establish a baseline of typical network behavior. When deviations occur, these systems can alert security teams to investigate potential breaches. This proactive approach allows organizations to address threats before they escalate.
Example: Vectra AI’s Cognito platform uses AI to analyze metadata across the network, detecting anomalies indicative of cyber threats such as insider attacks or external breaches.
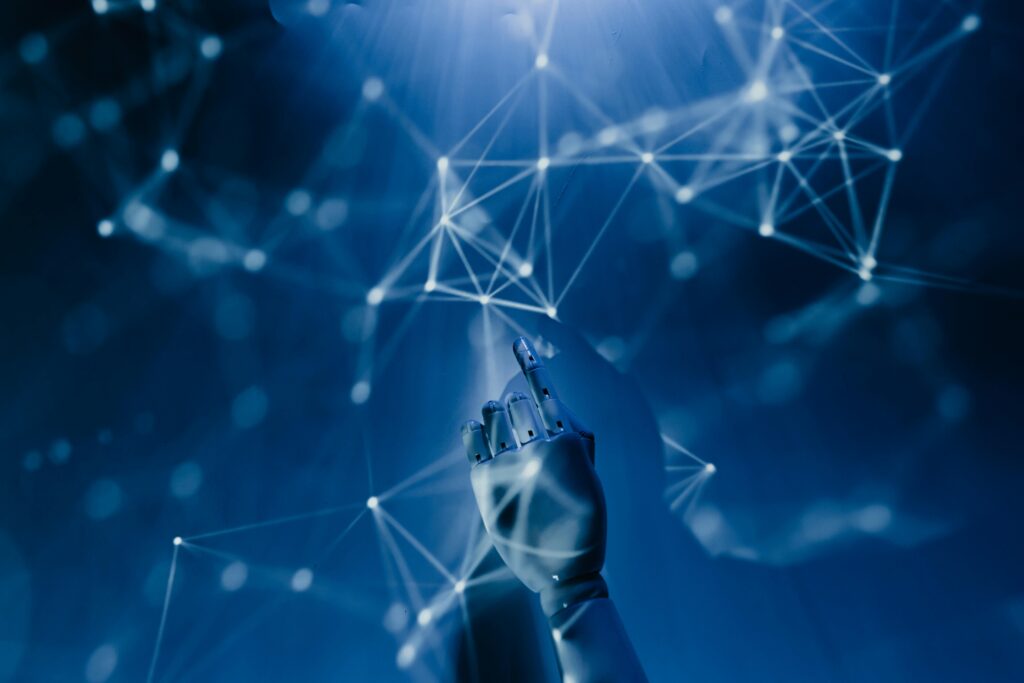
Challenges in Implementing AI and Machine Learning
1. Data Quality and Accessibility
For machine learning to be effective, organizations must have access to high-quality, comprehensive datasets. Inconsistent data formats and silos can hinder the development of accurate models. Ensuring that data is both accessible and of high quality is a critical challenge.
Solution: Organizations can invest in data management solutions that standardize and aggregate data from various sources, enabling more effective training of machine learning models.
2. Managing False Positives
One of the significant challenges in deploying AI in cybersecurity is the occurrence of false positives. AI systems can sometimes misinterpret benign activities as threats, overwhelming security teams and leading to alert fatigue. Striking the right balance between sensitivity and specificity is crucial for effective incident management.
Solution: Continuous tuning and refinement of AI algorithms, along with human oversight, can help minimize false positives while maintaining the ability to detect genuine threats.
3. Adaptation to Evolving Threats
The rapid evolution of cyber threats necessitates continuous updates to AI models. Organizations must be prepared to regularly retrain their systems with new data to maintain efficacy. Without ongoing learning, AI systems can become obsolete.
Solution: Establishing a regular schedule for model retraining, along with a robust feedback loop from security analysts, can ensure that AI systems remain effective against new threats.
4. Ethical Considerations and Governance
The integration of AI in cybersecurity raises important ethical issues, including privacy concerns and the potential for bias in algorithms. Establishing clear governance frameworks is essential to address these challenges and ensure responsible use of AI technologies.
Solution: Organizations should implement ethical guidelines for AI usage, conduct regular audits of AI systems for bias, and engage stakeholders in discussions about data privacy and security.
The Future Landscape of AI and Machine Learning in Cybersecurity
1. Integration with Existing Security Frameworks
As organizations recognize the value of AI and ML, they are increasingly integrating these technologies with their existing security infrastructures. This holistic approach leads to more cohesive and effective security strategies.
Example: Many organizations are adopting Security Information and Event Management (SIEM) systems that incorporate AI capabilities, allowing them to centralize security monitoring and incident response.
2. Collaboration Between AI and Human Experts
While AI can automate many processes, human expertise remains vital. The most effective cybersecurity strategies will involve a symbiotic relationship between AI systems and skilled professionals. Cybersecurity analysts will be tasked with interpreting AI-generated insights and making strategic decisions based on them.
Example: Organizations are increasingly training security teams to work alongside AI tools, enabling them to leverage technology for enhanced threat detection and response.
3. Evolution of Threat Intelligence Platforms
Future threat intelligence platforms will harness AI to provide actionable insights and real-time analysis, significantly improving decision-making and response strategies. These platforms will aggregate data from various sources, allowing organizations to identify and prioritize threats more effectively.
Example: Recorded Future combines AI-driven threat intelligence with human analysis, providing organizations with timely insights into emerging threats and vulnerabilities.
4. Regulatory Developments
As the use of AI in cybersecurity grows, so too will the need for regulatory frameworks that ensure ethical practices and accountability. Organizations must stay informed and compliant with these emerging regulations to avoid legal repercussions and ensure public trust.
Example: The European Union’s General Data Protection Regulation (GDPR) sets stringent guidelines for data privacy, impacting how organizations can utilize AI in cybersecurity and data protection.
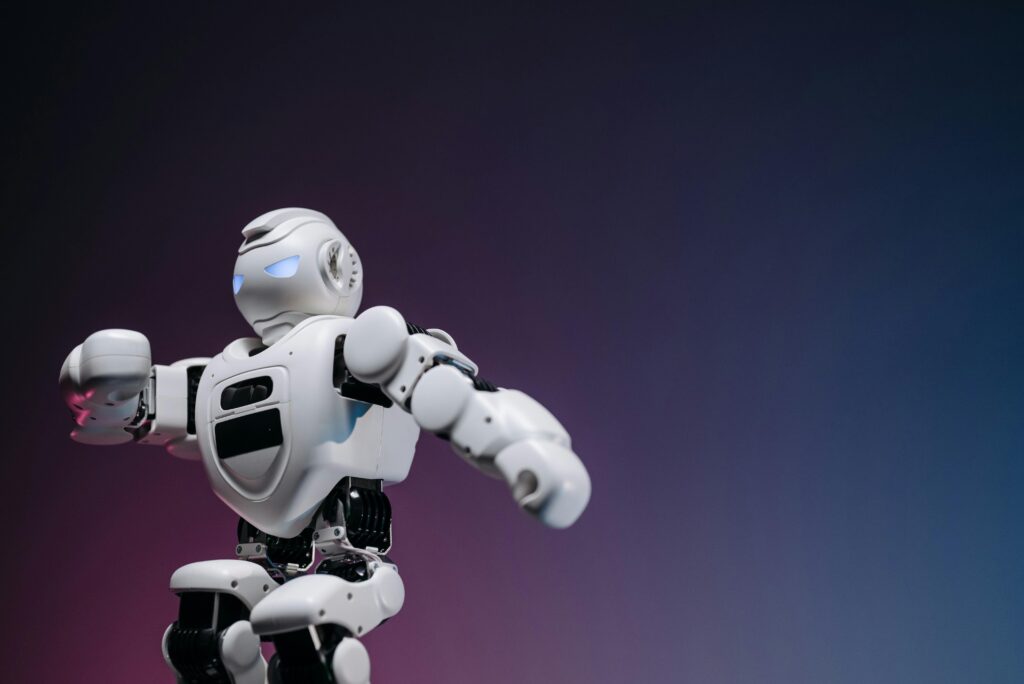
Conclusion
The integration of AI and machine learning into cybersecurity represents a paradigm shift in how organizations defend against cyber threats. By automating threat detection and response, leveraging predictive analytics, and improving overall security posture, these technologies offer a powerful toolkit for modern cybersecurity. However, successful implementation requires careful consideration of challenges such as data quality, ethical implications, and the need for continuous adaptation.
Organizations that prioritize the adoption of AI and machine learning technologies in their cybersecurity strategies will not only enhance their defenses but also position themselves as leaders in a rapidly evolving digital landscape. Investing in these advanced tools and fostering a culture of continuous learning will be key to safeguarding digital assets against the ever-growing threat of cybercrime.
Call to Action
To stay ahead of cyber threats, organizations must embrace the integration of AI and machine learning in their cybersecurity strategies. By investing in these technologies, training personnel, and fostering a proactive security culture, businesses can significantly enhance their defenses and create a safer digital environment for all.